Geocoding DoubleCheck: A Unique Location Accuracy
Assessment Tool for Parcel-level Geocoding
Initial release: August 2016
Geocoding is a process of converting an address (typically in the form of street number, street name, city, state and country) into a physical location on the globe (often expressed as latitude and longitude). It is one of the most popular spatial analysis techniques, and almost all major GIS software (e.g. QGIS, ArcGIS and MapInfo) and web mapping portals (e.g. Google Maps, Bing Maps and Apple Maps) provide geocoding tools and API options. But the big question is how reliable the geocoding result is from each geocoder.
Geocoding (location) accuracy is important for location-sensitive or location-centric business applications such as exposure management and risk analysis. Currently, very few assessment tools on this exist. Without the confidence on the location accuracy, subsequent analyses and results would be seriously compromised.
We have developed Geocoding DoubleCheck, a unique tool for assessing the location accuracy of the currently widely-used parcel-level geocoding. The project coverage here is 48 contiguous U.S. States.
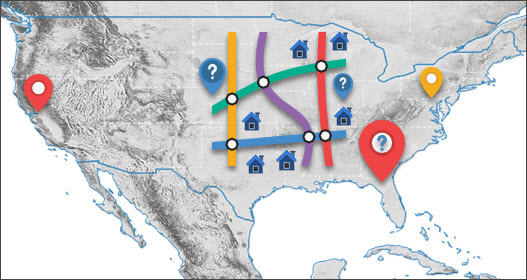
Parcel-level Geocoding vs. Building-level Geocoding
In earlier days, geocoding was realised by interpolating the range of address numbers specific to individual street segments (i.e. street-level geocoding). For advanced geocoding, the following two levels can be categorised (Figure 1):
Parcel-level geocoding: The geometric centroid of a land parcel (polygon) is used to represent the location of an address. This is a popular form of geocoding in some countries, e.g. about a half dozen private companies offering land parcel data with varying levels of completeness in the U.S. and the public release of Open Geocoded National Address File (G-NAF) in Australia.
Building-level geocoding: The geocoded location is indicated by the building footprint of a physical building and/or a point within (also known as rooftop-level geocoding). The latest pursuits are certainly geared towards this, but it needs significant investment if a study area is very large.
Obviously, for many location-centric applications, parcel-level geocoding is still not enough and what is required is the geocoding at the physical building level.
Figure 1: Comparison between parcel-level geocoding (left) and building-level geocoding (right). Location: Tompkins County, NY. (Acknowledgements: On this page, the sample land parcel and building footprint data are obtained from the Tompkins County GIS Division; high-resolution aerial imagery is from the USDA NAIP series.)
The difference between the two advanced geocoding levels above can be more easily appreciated if high-resolution aerial or satellite imagery is superimposed (Figure 2). Imagery provides a rich context and clearly shows various land covers. In this demonstration, the limitation of the parcel-level geocoding for location-centric applications such as risk mapping is obvious. For example, a house might be far away from adjacent fire-prone forest but its parcel centroid could be within forest. Figure 3 shows another example in risk mapping: a house might be located on a high ground 50m away from a nearby river but its parcel centroid could be in a very flood-prone zone.
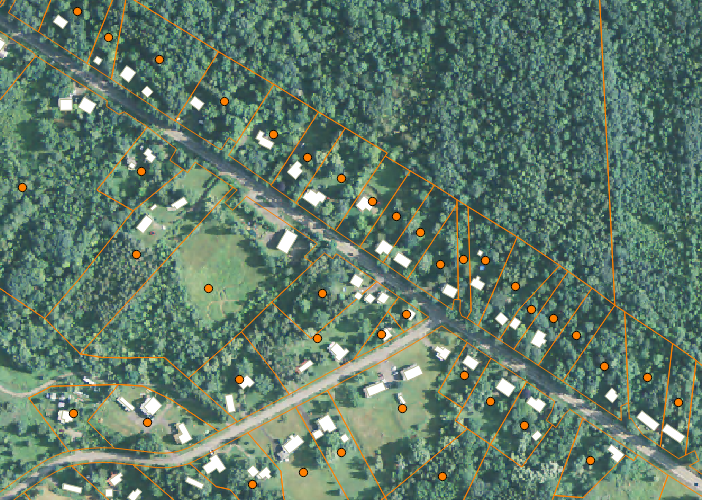
Figure 2: Land parcels and centroids (polygons and dots in orange) and building footprints (white polygons) are overlaid with
1m-resolution aerial imagery. The spatial discrepancy between parcel-level geocoding and building-level geocoding is shown.
Use of High-Resolution Imagery and Classified Vegetation
High-resolution imagery and classified vegetation are essential for the development of Geocoding DoubleCheck in assessing geocoding location accuracy (Figure 3). Ideally, high-resolution land cover maps can be sourced but they usually do not exist for a very large territory at this time. Figure 4 compares classified vegetation at 1m resolution and classified land covers from the 2011 National Land Cover Database (NLCD) at 30m resolution, and it is clear that for this type of application, the use of high-resolution imagery is a prerequisite.
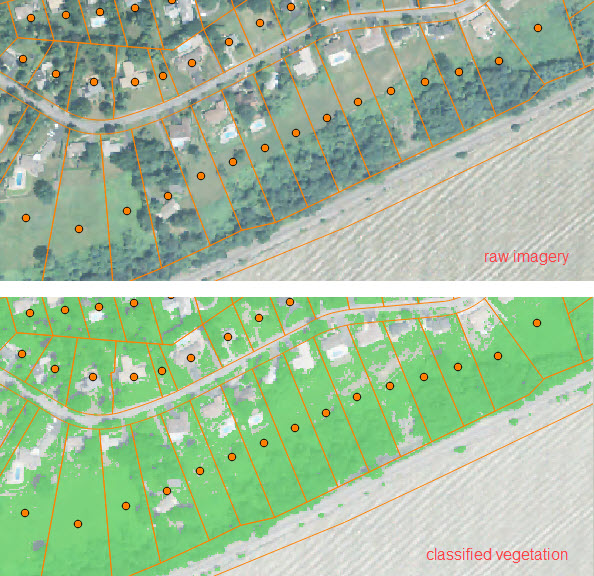
Figure 3: Parcel-level geocoding points superimposed with raw imagery (top) and classified vegetation (in green colour, bottom).
The classified vegetation map can be used to determine if the geocoded spot (parcel centroid) is on vegetation or not.
Figure 4: Comparison between classified 1m-resolution vegetation (left, in green) and the classified 30m-resolution land covers from NLCD 2011 (right, colours indicating different land covers). Land parcels (white polygons) and their geometric centroids (black dots) are superimposed.
Geocoding DoubleCheck takes advantage of the new, publicly-available high-resolution (1m) digital aerial imagery for 48 continuous U.S. States (from the USDA NAIP 2015/2014/2013 series) and the recently-classified unique vegetation dataset by BigData Earth (link). We are now able to determine if a geocoded location (i.e. land parcel centroid) is on vegetation or not. If it is on vegetation, the geocoded spot is subject to further location scrutiny or improvement. As the imagery we analysed is from agricultural growing seasons or with maximum “leaf on” conditions, the presence of vegetation is a universal feature and clearly signals non-building areas.
Application Example: Advancing Loss Modelling and Risk Mapping
We discuss two potential applications in relation to Geocoding DoubleCheck product: